SDG14: Life below Water
Ecosystem health assessment in Jiaozhou Bay, China(2019)
Scale: Local
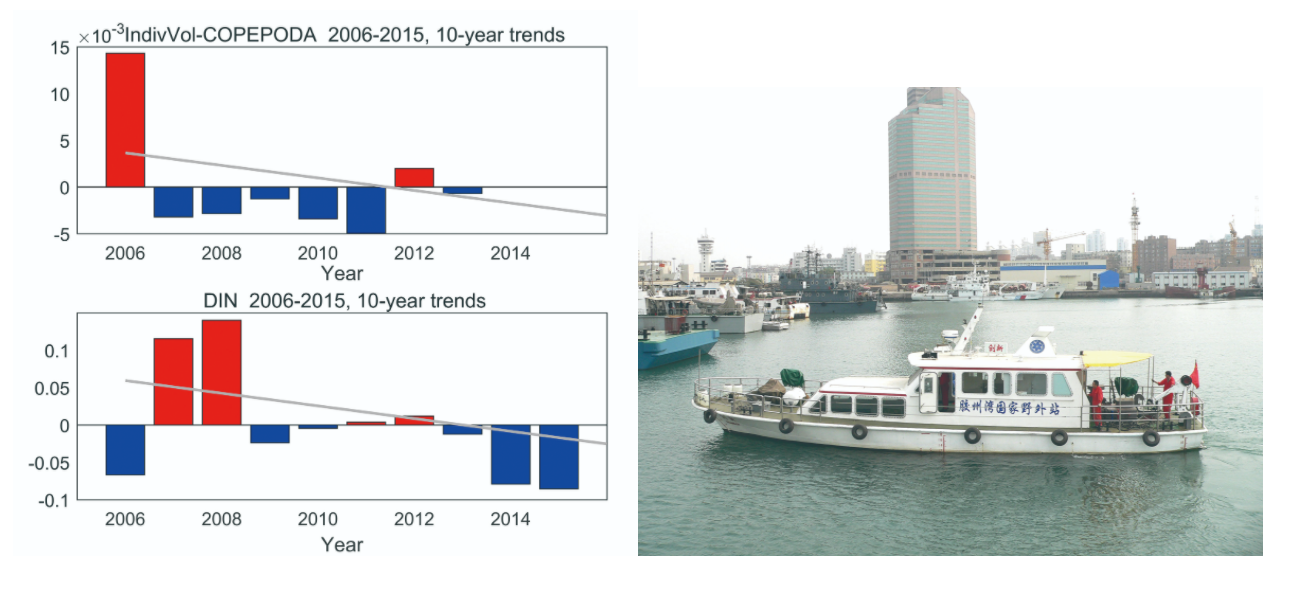
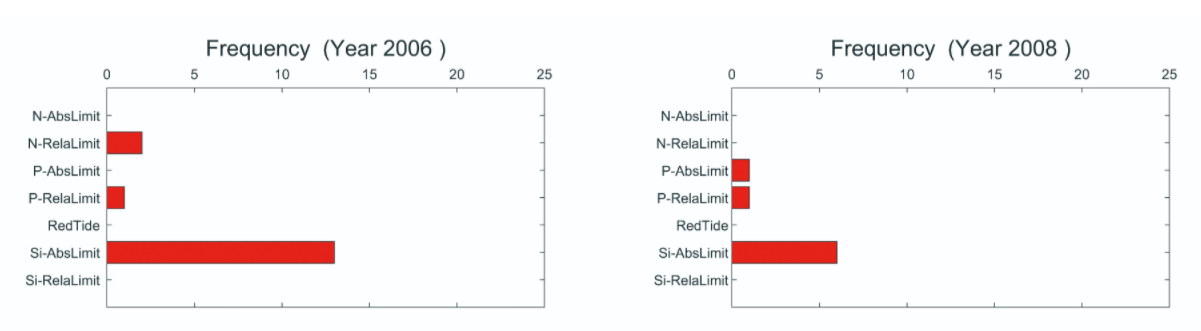
Study area: Jiaozhou Bay, China
One of the most important approaches to ensuring the protection and sustainable development of marine environments and resources is to establish ecosystem-based management practices that maintain a healthy ocean ecosystem. Marine ecosystem health assessments can be used as important decision support tools to provide direct, high-quality information and to guide sustainable coastal use and development. It can also help to improve an ecologically sound management strategy for sustainable use and development of coastal areas. Industrialization, urbanization, aquaculture, agriculture, tourism, and other human activities, combined with global changes, are compounding pressures on coastal ecosystems. Ecosystem health assessment needs to integrate relevant data sources from different aspects describing ecosystem conditions and impacts of existing pressures. A coordinated way of integrating land and marine data/information is hence necessary to assess current cumulative pressures and impacts. The research is working to develop new approaches based on multi-source data, big data analysis, and machine learning technologies.
Target 14.2: By 2020, sustainably manage and protect marine and coastal ecosystems to avoid significant adverse impacts, including by strengthening their resilience and take action for their restoration, to achieve healthy and productive oceans.
Indicator 14.2.1: Proportion of national exclusive economic zones managed using ecosystem-based approaches.
Method
Taking Jiaozhou Bay as a case study, a primary assessment framework was established based on long-term studies that focused on variations in meteorological, hydrological, chemical, and biological elements and key processes, as well as their impacts on marine ecosystem evolution. With a focus on SDG 14.2, a selection of indicators and guideline settings were reviewed to gain a better understanding of ecosystem structure,2006.01-2015.12, observation data in Jiaozhou Bayincluding: phytoplankton, zooplankton, benthos, bacteria, from 2006-2015 environmental situation bulletins.hydrological, physical, and chemical factors.
Data used in this case
services, functions, and ecological disasters and diseases. The present case study uses data mining to improve guidelines, thresholds, and reference settings in existing health assessments using machine learning techniques. The goal is to translate monitoring, observation, and research results into information that can be understood easily by the public and policy makers.
Aquaculture production, area, and other environmental data from 2006-2015 environmental situation bulletins.
Results and analysis
The 2006-2015 long-term variations in the meteorological, hydrological, chemical, and biological elements at Jiaozhou Bay showed that the marine ecosystem in the bay was experiencing a change. Nutrient concentrations in the bay exhibited a decreasing trend, suggesting that the water quality in the bay has been improving; while the health conditions of the plankton community, as represented by the phytoplankton/zooplankton community compositions and size distributions, exhibited an ascending trend (Figure 1). The long-term changes in nutrient concentration and structure led to an increasing number of nutrient limitations in the bay (Figure 2), which has potential impacts on phytoplankton communities and water quality.
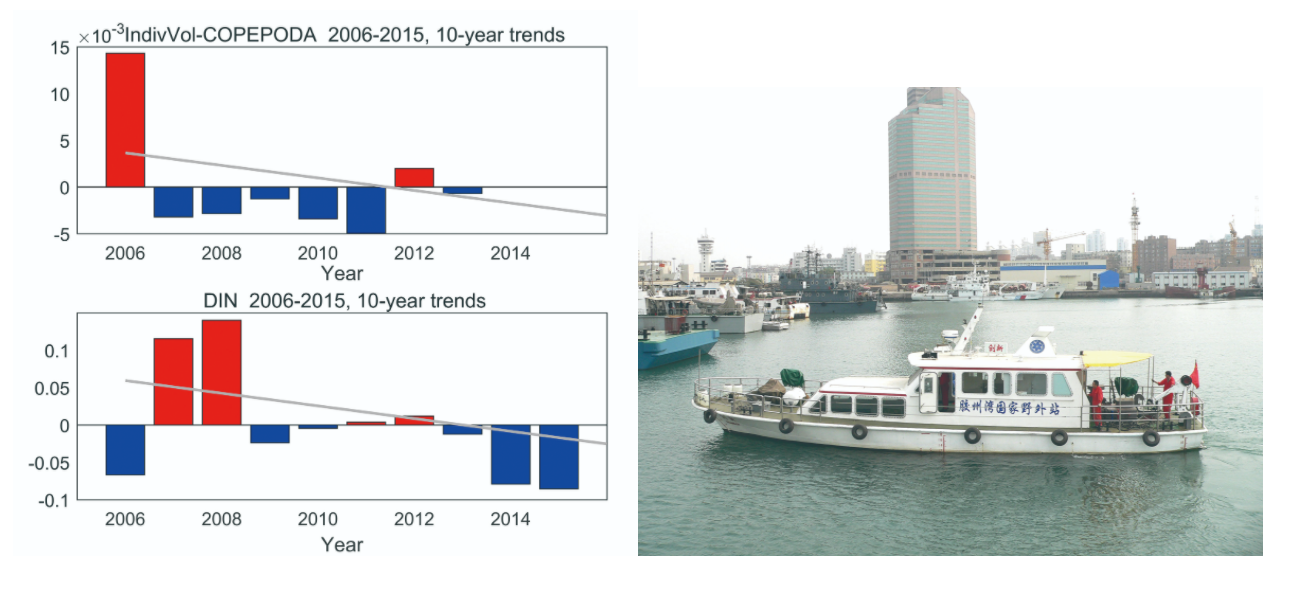
Figure 1. Variations in plankton abundance and nutrient concentration in Jiaozhou Bay from 2006 to 2015.
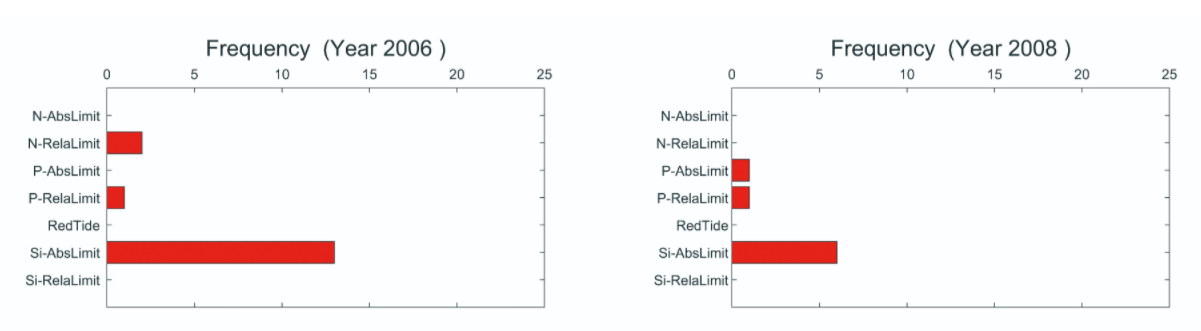

Figure 2. Frequencies of nutrient limitations and ecological disasters in Jiaozhou Bay for 2006, 2008, 2012, and 2015.
The results of the pilot health assessment in Jiaozhou Bay show that the health condition of the Jiaozhou Bay ecosystem is classified as grade “B”,which means that the ecosystem is in relatively good condition. Environmental conditions during the study duration show an improving trend, consistent with the varying trend in nutrients. The biota condition in the bay had a slight declining trend, similar to the plankton community condition indicators. The assessment results show nonlinear characteristics in the long-term variations of indicators at different levels, partly consistent with historical trends, with only a few indicators showing a different trend over the past decade.
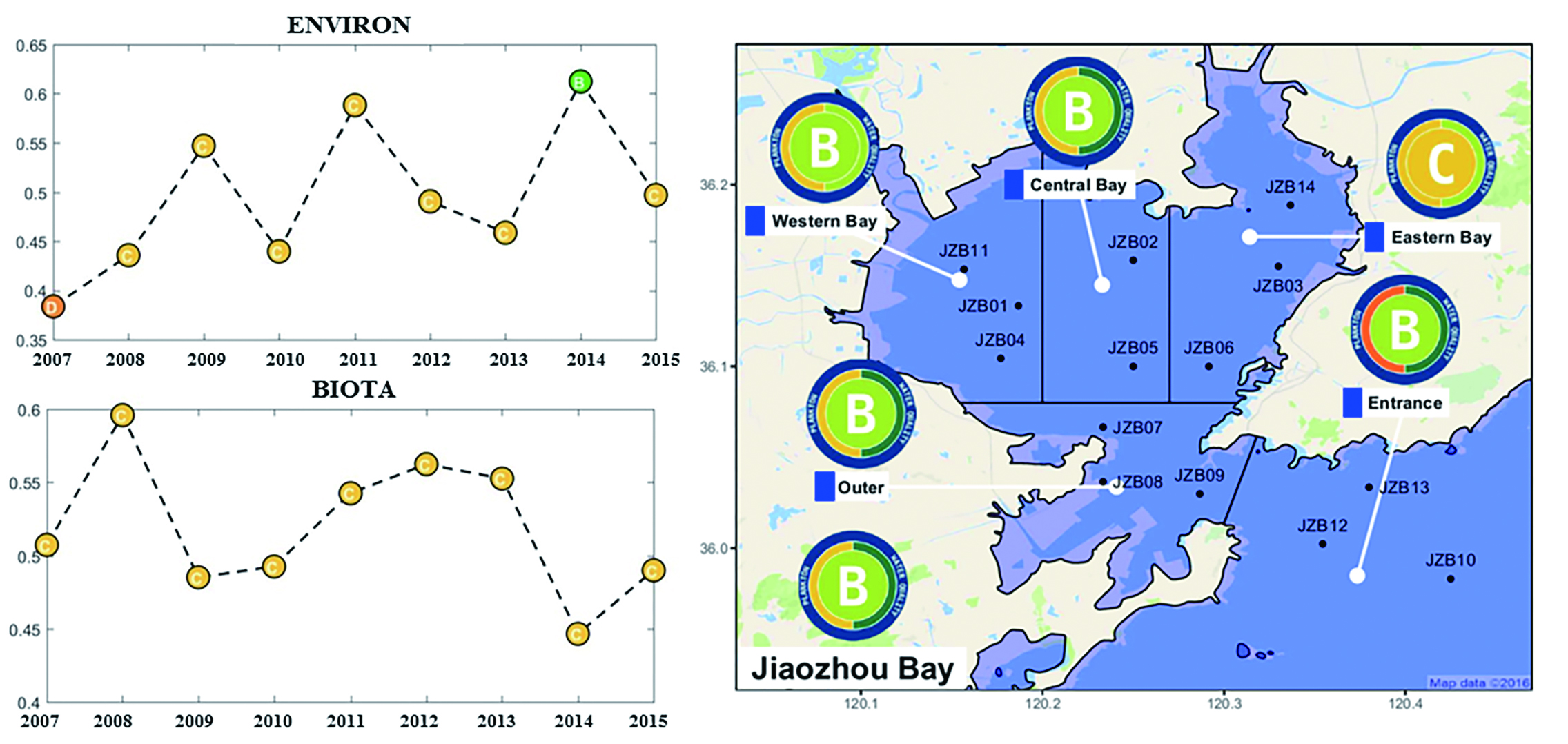
Figure 3. Evolution of marine ecosystem conditions from 2007 to 2015, and result of pilot health assessment in Jiaozhou Bay.
Highlights |
The case study improved ecosystem health assessment research by reviewing a selection of indicators and guideline settings to gain a better understanding of ecosystem structure, services, functions, and ecological disasters and diseases. By applying machine learning-based data mining technology, the guideline, threshold, and reference settings in existing health assessments can be improved. The improved framework was used to conduct a pilot marine ecosystem health assessment in Jiaozhou Bay. The overall health condition of Jiaozhou Bay is relatively good: environmental conditions during the study duration show an improving trend, and biota conditions in the bay had a slight declining trend. The assessment results show nonlinear characteristics in the long-term variations of indicators at different levels, partly consistent with historical trends, with only a few indicators showing a different trend over the past decade. |
Outlook
The current case study improves existing coastal ecosystem health assessment research in the following aspects: selecting indicators from ecosystem structure and changes; establishing reference and guideline values for indicators using machine learning technology; and improving assessment methods according to data types and characteristics.
The marine ecosystem health assessment will be further developed, including diagnostic models and scenario simulation models to analyze stress factors on marine health and to diagnose marine ecosystem health. By integrating diagnostic, water quality, ecological, and hydrodynamic modules into a model platform, it will be able to simulate different scenarios and predict possible responses of the marine ecosystem.
This structured research methodology will be repeated to study other coastal regions and improve our model for a wide variety of environments. With collaborating partners such as the Australian Institute of Marine Science and the Indonesian Institute of Sciences, the current research is being considered for application to other areas.
Advisory reports as decision support tools will be developed with the aim to support national strategic objectives and to facilitate targeted end-users towards restoration and protection of coastal and marine environments.